1. 案例介绍
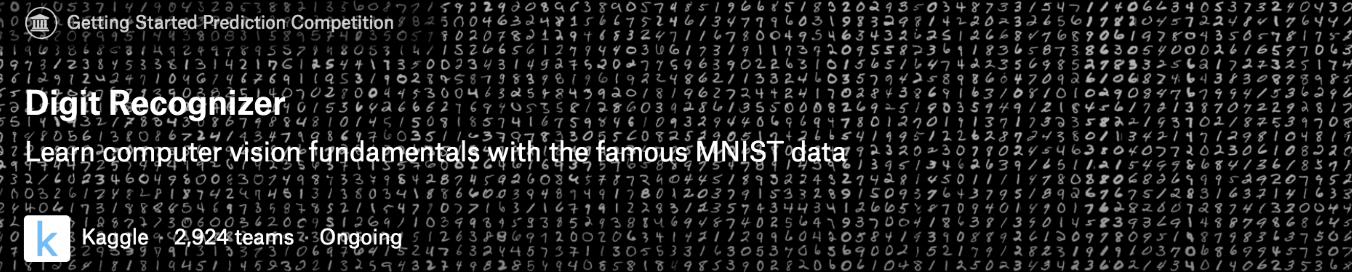
MNIST手写数字识别 是计算机视觉领域中 “hello world”级别的数据集
- 1999年发布,成为分类算法基准测试的基础
- 随着新的机器学习技术的出现,MNIST仍然是研究人员和学习者的可靠资源。
本次案例中,我们的目标是从数万个手写图像的数据集中正确识别数字。
2. 数据介绍
数据文件 train.csv 和 test.csv 包含从 0 到 9 的手绘数字的灰度图像。
- 每个图像高 28 像素,宽28 像素,共784个像素。
- 每个像素取值范围[0,255],取值越大意味着该像素颜色越深
- 训练数据集(train.csv)共785列。第一列为 “标签”,为该图片对应的手写数字。其余784列为该图像的像素值
- 训练集中的特征名称均有pixel前缀,后面的数字([0,783])代表了像素的序号。
像素组成图像如下:
000 001 002 003 ... 026 027
028 029 030 031 ... 054 055
056 057 058 059 ... 082 083
| | | | ...... | |
728 729 730 731 ... 754 755
756 757 758 759 ... 782 783
数据集示例如下:
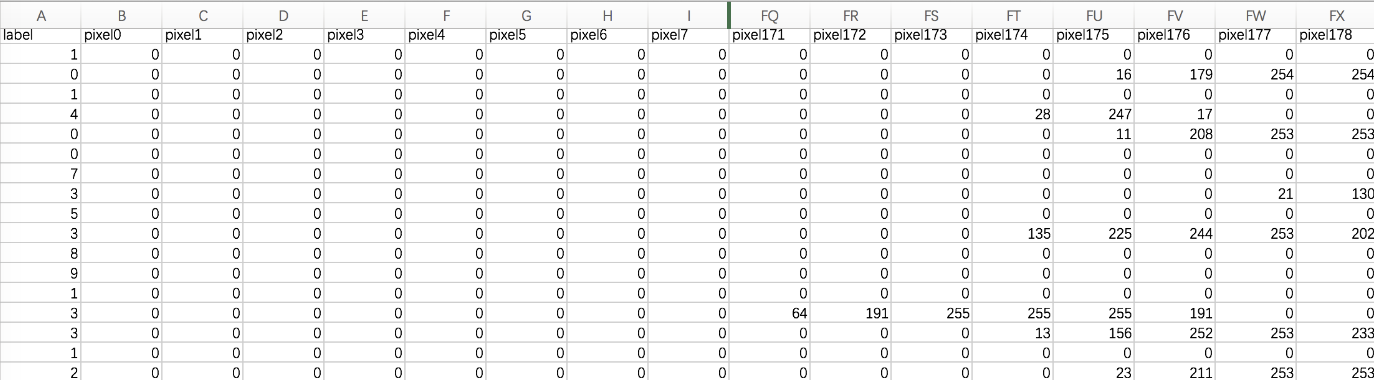
3. 示例代码
import matplotlib.pyplot as plt
import pandas as pd
from sklearn.model_selection import train_test_split
from sklearn.neighbors import KNeighborsClassifier
import joblib
from collections import Counter
def show_digit(idx):
# 加载数据
data = pd.read_csv('data/手写数字识别.csv')
if idx < 0 or idx > len(data) - 1:
return
x = data.iloc[:, 1:]
y = data.iloc[:,0]
print('当前数字的标签为:',y[idx])
# data 修改为 ndarray 类型
data_ = x.iloc[idx].values
# 将数据形状修改为 28*28
data_ = data_.reshape(28, 28)
# 关闭坐标轴标签
plt.axis('off')
# 显示图像
plt.imshow(data_)
plt.show()
def train_model():
# 1. 加载手写数字数据集
data = pd.read_csv('data/手写数字识别.csv')
x = data.iloc[:, 1:] / 255
y = data.iloc[:, 0]
# 2. 打印数据基本信息
print('数据基本信息:', x.shape)
print('类别数据比例:', Counter(y))
# 3. 分割数据集
split_data = train_test_split(x, y, test_size=0.2, stratify=y, random_state=0)
x_train, x_test, y_train, y_test = split_data
# 4. 模型训练
estimator = KNeighborsClassifier(n_neighbors=3)
estimator.fit(x_train, y_train)
# 5. 模型评估
acc = estimator.score(x_test, y_test)
print('测试集准确率: %.2f' % acc)
# 6. 模型保存
joblib.dump(estimator, 'model/knn.pth')
def test_model():
# 读取图片数据
import matplotlib.pyplot as plt
import joblib
img = plt.imread('temp/demo.png')
plt.imshow(img)
# 加载模型
knn = joblib.load('model/knn.pth')
y_pred = knn.predict(img.reshape(1, -1))
print('您绘制的数字是:', y_pred)
if __name__ == '__main__':
# 显示部分数字
show_digit(1)
# 训练模型
train_model()
# 测试模型
test_model()